Making our Jetson Nano Speak - Text To Speech
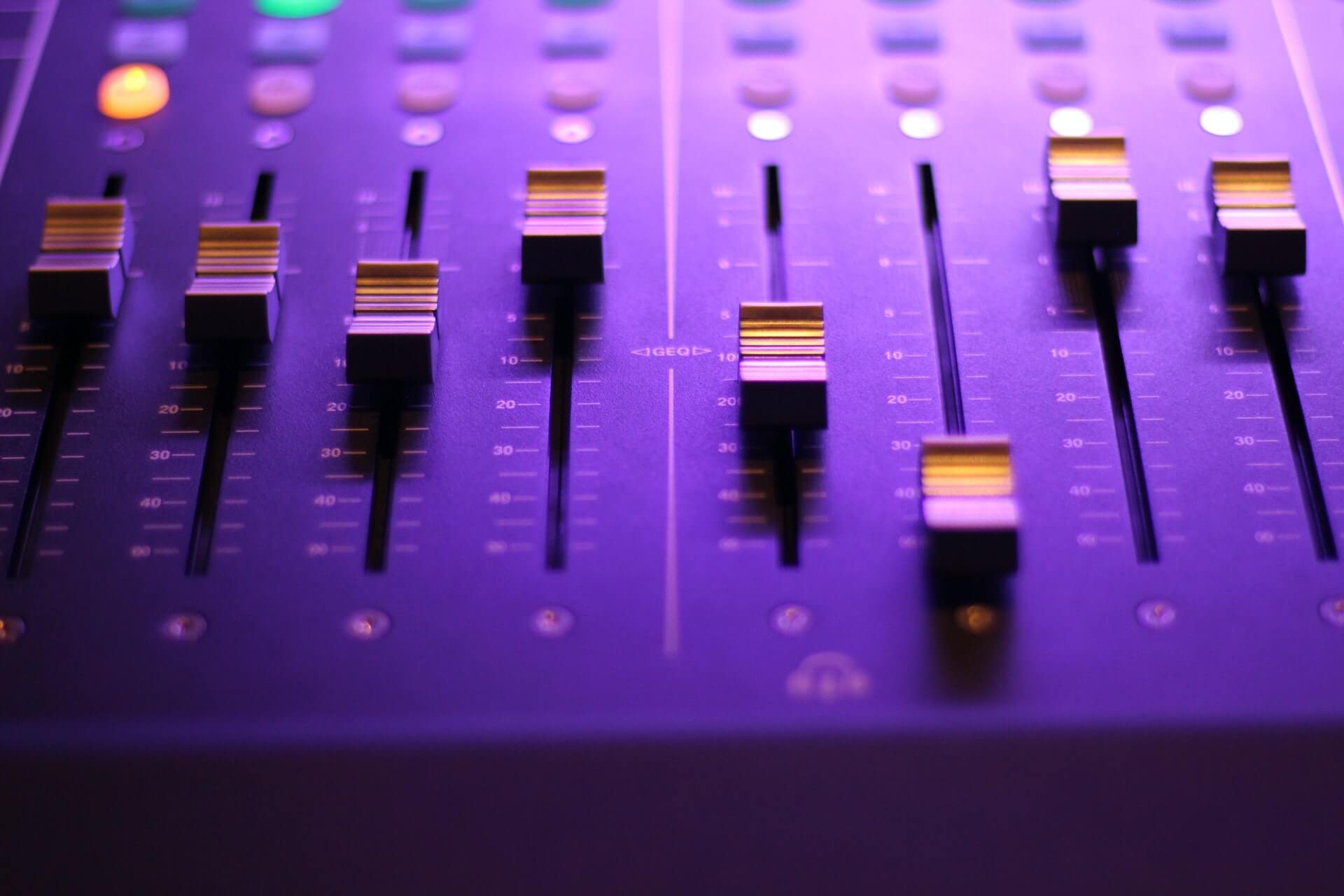
Photo by Denisse Leon on Unsplash
Making our Jetson Nano Speak - Text To Speech⌗
Today I will be covering how (I tried) to convert text to speech on my Jetson Nano running ROS. And what I learned from the experience.
Text To Speech Process⌗
The process to convert text to speech is as follows, you encode your input text, feed encoded text into a model that can generate Mel Spectograms (e.g Tacotron 2) and finally feed those Mel Spectograms into a model that can generate synthesized audio from it (e.g. WaveGlow) i.e.
Text
-> Encoded Text
-> Tacotron 2
-> WaveGlow
[trained on LJSpeech
or CMU ARCTIC
for instance]
Below is an example sequence diagram for a robot running ROS, being used to generate audio.
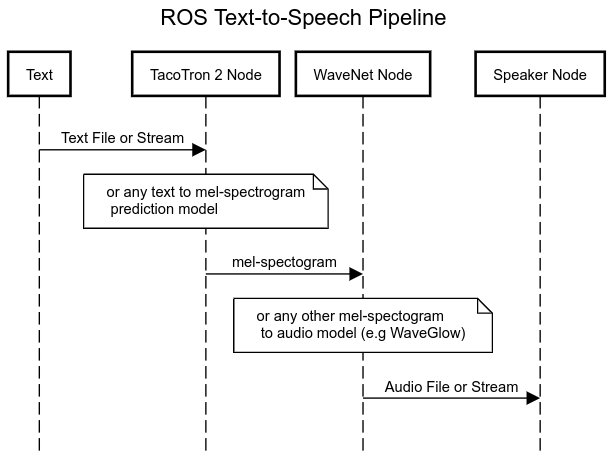
Tacotron 2 and WaveGlow
The Tacotron 2 and WaveGlow model form a text-to-speech system that enables user to synthesise a natural sounding speech from raw transcripts without any additional prosody information. The Tacotron 2 model produces mel spectrograms from input text using encoder-decoder architecture. WaveGlow (also available via torch.hub) is a flow-based model that consumes the mel spectrograms to generate speech - source nvidia deep learning examples tacotron2
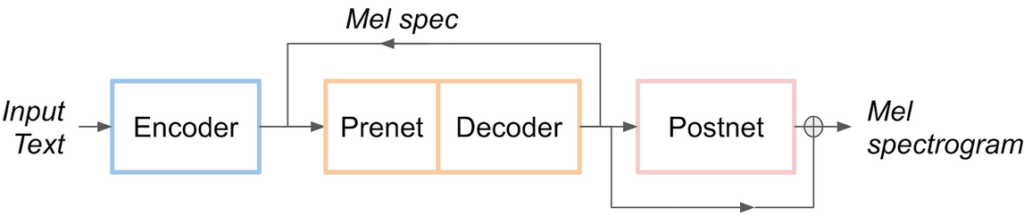
I thought this would be straight forward but this took me down the road of dependency hell and understanding the limitations of the Jetson Nano!
Dependency Hell⌗
First attempt: Download pre-trained Tacotron 2 and WaveNet then convert both to ONNX (ended in failure)
Initially I had to install libllvm
using this amazing guide by jefflgaol.
Then I changed my /etc/apt/sources.list
to
## Removed all but the new repository
deb http://ports.ubuntu.com/ubuntu-ports/ eoan main universe
So I could install the latest supported version of libtbb-dev
.
I installed ONNX
sudo -H pip install protobuf
sudo apt-get install protobuf-compiler libprotoc-dev
sudo -H pip install onnx
Installed sklearn
sudo apt-get install python-sklearn
sudo apt-get install python3-sklearn
Keep in mind when I say install
it means I had to build AND install the libraries on the nano which was very slow!
I also had to install the following python dependencies from here so the model could even load:
libffi-dev
libssl-dev
librosa
unidecode
inflect
libavcodec-dev
andlibavformat-dev
libswscale-dev
- Nvidia’s
dllogger
pycuda
, for this I had to use someones custom installation script!pytorch v1.5.0
andtorchvision v0.6.0
from here (this was a very long build and also finding the information to install that specific version was tedious)- Nvidia’s
apex
After building and installing all the above, I tried to convert the model to ONNX but some layers in the model were Not Supported by ONNX. Damn…
Second attempt: using code provided on the DeepLearningExamples repo (also ended in failure)
I downloaded NVIDIA/DeepLearningExamples and used the provided script to try and export the pre-trained model I had gotten in the first attempt.
- The commands to actually export are
cd ~/Code/DeepLearningExamples/PyTorch/SpeechSynthesis/Tacotron2
python3 exports/export_tacotron2_onnx.py --tacotron2 ./model_checkpoints/nvidia_tacotron2pyt_fp16_20190427 -o output/ --fp16
python3 exports/export_waveglow_onnx.py --waveglow ./model_checkpoints/nvidia_waveglow256pyt_fp16 --wn-channels 256 -o output/ --fp16
When I ran this, my jetson was simply ran out of memory, this is a lost cause
Sound generation on the desktop (third attempt?)⌗
I first tried to download and train the models using my CPU, that was a foolish idea, so I bought a GPU and it performs alot better
- 4GB GTX 1650 with CUDA 11.0 (pytorch 1.5 currently uses CUDA 10.2, though it still works as pytorch ships with its own CUDA version)
- Memory is a problem but that can be solved with smaller batch sizes e.g (batch_size=1)
- Training is ALOT faster 10x faster at least
My currenty workstation is in a hybrid configuration
- AMD 7870 for normal operations (display/sound)
- GTX 1650 solely for training Models
- It was hard to setup (tons of driver issues) but worth it
Here is where we get onto some (okay, a little) progress and here is where I realize that Generative Networks have very high system requirements!
I began training WaveNet using pytorch-wavenet by vincentherrmann
on GitHub
I did not even train one complete epoch after leaving my PC on overnight, got to 150k iterations though…
- keep in mind, although the GPU did not perform optimally with WaveGlow, it performed flawlessly when doing transfer learning with images
When generating music, generating 1 second (16000 samples) of sound ate about 16gb of memory… (though I believe this memory issue can be solved by streaming the data onto a file rather than naively holding it in memory)
Notes / Lesson’s Learned⌗
Do NOT do ML training on your embedded device (e.g jetson nano)
-
Do it on a Regular PC with a GPU (if you have one)
-
you can use a software suite like conda
-
Even better, if you don’t have a PC with a GPU, use a cloud service with a GPU like AWS P2 or P3 as this is Easier, Quicker and Cheaper
- Example AWS P2 (cheapest $0.9/hr) or P2 Spot Instance (cheapest $0.2/hr)
-
Attach an AMI (Amazon Machine Image i.e a VM) with all our DL tools installed
- Search for “Amazon Deep Learning AMI”
-
Attach EBS for persistence
-
- Example AWS P2 (cheapest $0.9/hr) or P2 Spot Instance (cheapest $0.2/hr)
Only run tensor rt
optimization and models on the embedded device!
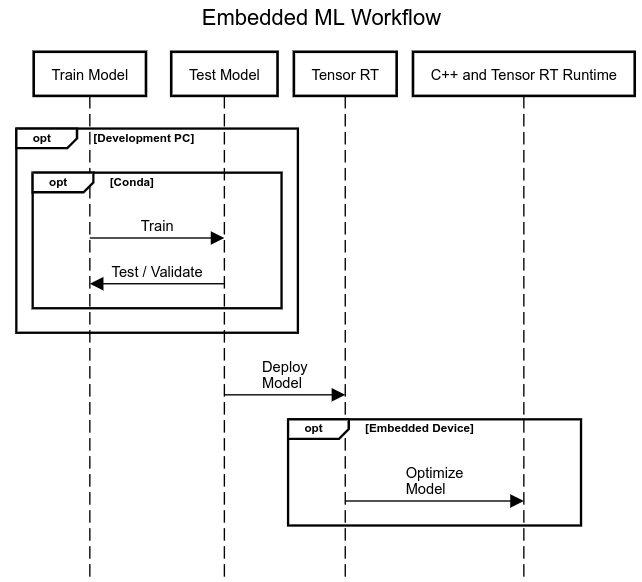
The jetson nano cannot handle large generative networks, especially one geared towards complex data types like sound (e.g Wavenet)
Alot of open source models are implemented using pytorch, so I will be using pytorch models directly in C++ rather that using tensor rt for now…
Going forward my new development practice will be⌗
-
Develop, Test and Convert model on Main PC using conda (e.g. Pytorch to ONNX model)
- Training, Testing, Converting etc. models on the jetson nano is NOT feasible!
-
Send model to Embedded device (e.g. jetson nano)
-
Optimize model with Tensor RT on Embedded device
- Or Convert to Torch Script via Tracing
-
Run model with Tensor RT Runtime on Embedded device
- or run with LibTorch (Pytorch C++ API)
-
Develop for ROS C++ on the Embedded device
Pheeeeeeeeeeeeeew, I hope you enjoyed this dreary tale and I also hope you learned from my mistakes!
Next time we are going to get into Reinforment Learning and legged robots.
I will see you then!
Sources / Recommended Reading⌗
-
How to Deploy Real-Time Text-to-Speech Applications on GPUs Using TensorRT
-
Find Nvidia’s WaveGlow and TacoTron 2 implementation on their GitHub, look in
PyTorch/SpeechSynthesis/Tacotron2/
-
pytorch-wavenet by
vincentherrmann